GREETING
Director of the Center for Artificial Intelligence Research in Therapeutics,
Professor of Artificial Intelligence Medicine, Graduate School of Medicine,
Chiba UniversityEiryo Kawakami
Eiryo Kawakami
To date, there have been three booms in artificial intelligence (AI). The first two booms ended without a breakthrough, due to the lack of theoretical support, lack of technical processing power of computers, and lack of data. However, AI has received significant attention in the third boom that has continued from 2006 to the present. The triggers of the third boom are big data and deep learning. Today’s AI system is quite different from previous computing systems, in that AI can learn patterns and rules from a large amount of data without being taught by humans, and is continuing to make innovations in all fields of modern society.
Can we also make this innovation in the medical field? Can we create a new academic field, “AI Therapeutics,” by integrating AI with traditional medical disciplines? This Center was established in April 2018 by Dr. Takeshi Tokuhisa, President of Chiba University and Dr. Toshinori Nakayama, Dean of Graduate School of Medicine, Chiba University, in the earnest hope of applying AI research above all else to real-world clinical practice as soon as possible and creating new therapies. Later, I took office as Director of the Center in January 2019, and up to the present.
The most important objective of AI research in healthcare is not to develop AI itself, but to develop AI to “solve the challenges of clinical and basic medical sciences.” For this purpose, we are committed to the development of AI by forming an appropriate team after interviewing thoroughly with clinical physicians and basic medical scientists and setting the challenges. What we are very conscious of in this process is open innovation. In previous medical research, there has been a strong sense of sectionalism among different professor offices, with little collaboration across scientific fields and domains. For medical research using AI and data science, however, the barriers of sectionalism need to be removed to the extent possible, and various scientists from basic medical science, clinical medicine, mathematics, information science, etc. are brought together to create something new. Our theme is to address AI therapeutics from all perspectives and advance medical science itself.
In terms of the development of medical science, the fostering of young scientists is also a reason for being of this Center. It is out role to lay the foundation for young people, who are living and learning in an environment where AI is taken for granted, to be able to play an active role in the medical field in the future. As the Director of the Center, I would like to coordinate the skills and experiences of each scientist, so that they can be connected to make a network. I hope that this will ultimately help make our healthcare and society better.
MORE
INTRODUCTION
DIVISIONS & MEMBERS
Clinical Research Division
Medical Immunology Shinichiro Motohashi
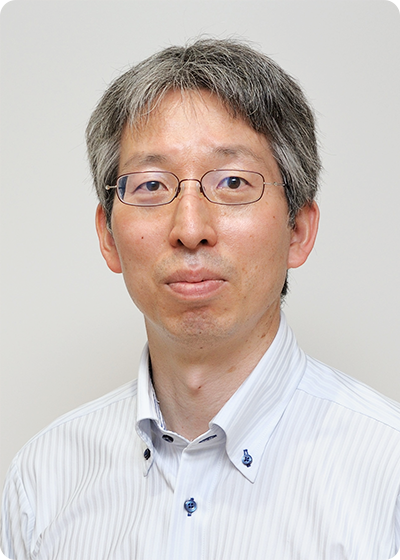
Shinichiro MotohashiProfessor, Department of Medical Immunology, Chiba University
- Shinichiro MotohashiProfessor, Department of Medical Immunology, Chiba University
-
In the field of tumor immunology, we are trying to establish a new immunotherapy for cancer. To utilize the vast amount of data from gene expression analysis and proteome analysis, we are trying to find the truth behind natural phenomena using a variety of experimental techniques. We have, for example, induced pluripotent stem cell-derived natural killer T (iPS-NKT) cells that can kill cancer cells. How iPS-NKT cells act and behave in the body will be elucidated by analyses using techniques, such as AI.
The Clinical Research Division of our Center is conducting interventional clinical trials to verify the effectiveness of new therapies. For example, if a drug showed a very high inhibitory effect in 20% of lung cancer patients, it means, conversely, that the drug was not effective in the remaining 80% of the patients. Then, how can we create a drug that is effective for all patients? At what point was the antitumor effect of the test drug inhibited?
In our department, we are studying this area clinically by an interventional study. More specifically, we collect blood and other biological samples from many patients and analyze them comprehensively.Today, we are still conducting clinical trials by dividing subjects into the “intervention group” and the “control group” to test a new treatment. However, if various data are accumulated and processed by AI in the future, clinical trials without a “control group” may be established as valid. Although this may not be realized anytime soon, I expect that our Center will become a place where people engaged in next-generation clinical research will be able to play a more active role.
Diagnostic Pathology Junichiro Ikeda
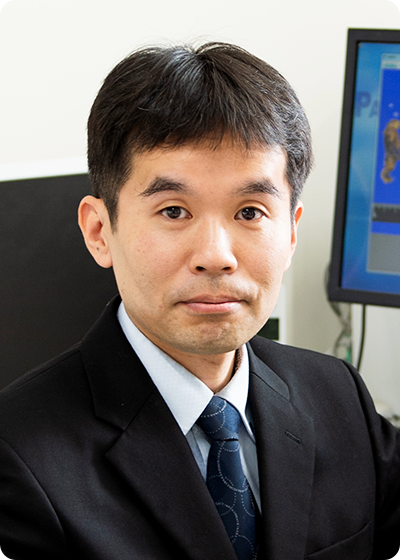
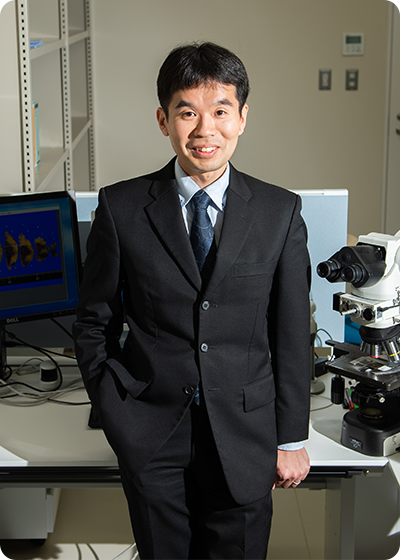
Junichiro IkedaProfessor, Department of Diagnostic Pathology, Chiba University
- Junichiro IkedaProfessor, Department of Diagnostic Pathology, Chiba University
-
Diagnostic pathology I am specializing in is a specialty where pathological specimens are prepared from samples collected from patients during examination or surgery, and a diagnosis is made using a microscope. We are not only making a pathological diagnosis, but also performing morphological observations, to find various characteristics and elucidate the pathological mechanism. In recent years, many attempts have been made worldwide to elucidate the pathological mechanism by making AI read pathological images and perform deep learning. We are also planning to make it possible to classify characteristic genetic abnormalities without genetic testing, by using both pathological images and AI, in cooperation with the Department of Breast Surgery.
The role I play in the Clinical Research Division of our Center is to appropriately store pathological specimens and images. Recently, there have been significant advances in the methods of comprehensively investigating various genes, and the search for genetic abnormalities that may contribute to actual treatment is also performed using pathological specimens. For example, the same cancer can be seen in different forms, depending on the patient. I would like to classify pathological images and determine what drug is likely to be effective against genetic abnormalities one by one by using pathological images and AI .
We have recently been engaged in the development of software to support pathological diagnosis by using AI. The number of pathologists is limited, and there is often only one pathologist in one hospital. In such cases, there is no person who indicates a careless oversight or misdiagnosis if it occurs, and it would be a serious problem in the quality control of health care. I wish to reduce the burden on pathologists by enabling them to share their roles with AI. Because of the characteristics of each organ, it will take a long time before software that can diagnose many diseases is created and put into practical use. The Japanese Society of Pathology has recently taken the initiative and started collecting and analyzing cases of diseases of the stomach, lung, and mammary gland. We are working with Med-Tech Link Center to detect lymph node metastasis in gastric cancer by using AI. We will make further efforts to make the pathological diagnosis of all diseases feasible, as soon as possible.
Translational Omics Division
Future Medicine Education and Research Organization Osamu Ohara
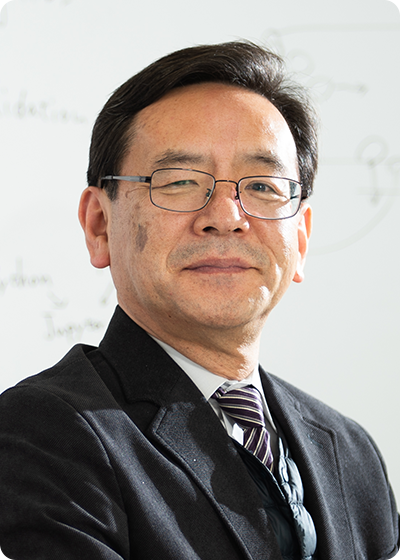
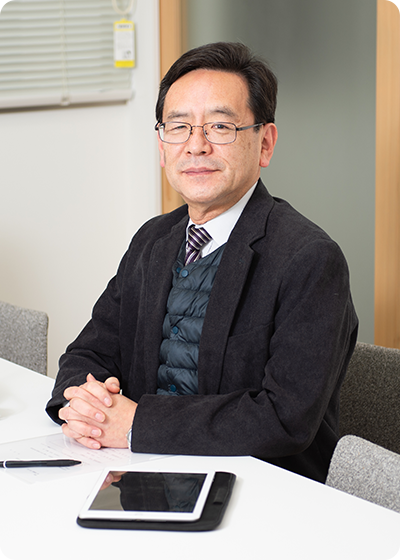
Osamu OharaSpecially Appointed Professor, Future Medicine Education and Research Organization at Chiba University
Deputy Director of Kazusa DNA Research Institute and other departments
- Osamu OharaSpecially Appointed Professor, Future Medicine Education and Research Organization at Chiba University
Deputy Director of Kazusa DNA Research Institute and other departments -
Cells in a living organism have essentially the same genome or “genetic blueprint,” but cells we can see are different from each other in appearance. This is because the way of reading the same blueprint differs among different cells. Proteins are produced from the translated part of the genome, and different cells are formed, depending on the nature of the proteins. The role of the Translational Omics Research Division is to investigate the amount and type of proteins in cells or body comprehensively, and to determine the molecular profiles that reflect the state of the biological system. We are accumulating basic data using clinical materials to help promote clinical research.
There are about 20,000 protein blueprints in the human genome. It has become possible to comprehensively determine the amount and type of molecules derived from such genomic information. However, if we try to comprehensively acquire data on molecular profiles in the body, there will be a deluge of information, making it impossible for humans to extract their meanings. Therefore, it is necessary to translate measurement data, by using AI, into medically or scientifically meaningful information. To improve the precision of processing by AI, we will accumulate basic data necessary for AI to learn, at our division.
The 21st century is an era in which we must confront various problems derived from complex systems. We need to have the wisdom of choosing the best way at the time and the place. The same is true for the economy and for the human body. In the future, AI used for healthcare may provide hints to stabilize the economy. Conversely, AI used for the economy may provide hints about new therapies in healthcare. To foster AI that will become our good partner, it is our role to perform experiments and verifications, one by one, from which we aim to accumulate achievements in solving problems.
Basic Research Division
Molecular Oncology Atsushi Kaneda
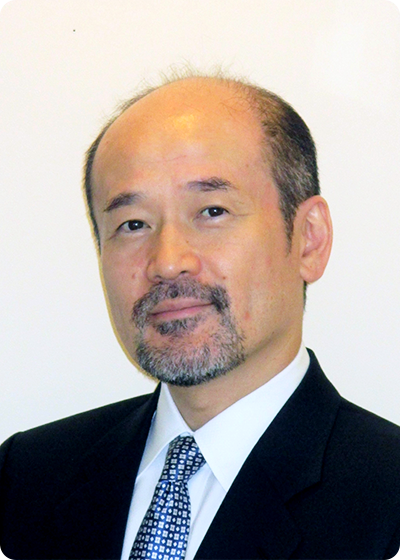
Atsushi KanedaProfessor, Department of Molecular Oncology, Graduate School of Medicine, Chiba University
- Atsushi KanedaProfessor, Department of Molecular Oncology, Graduate School of Medicine, Chiba University
-
As a basic research department of our Center, we provide a large amount of data for use in machine learning. First, we would like to analyze molecular biological data on cancers and tumors, which are main diseases under study in our field. In addition, we would like to comprehensively collect and analyze data on the epigenome, which is an approach to research in our field, to investigate how the epigenome, the set of chemical modifications to the genome, regulates gene expression on the genome. If we use next-generation sequencers, we can analyze not only cancer but also all diseases and life phenomena in the same way. We also wish to contribute to acquiring and providing a large amount of data on research samples collected in other fields.
What we expect from AI in the Basic Research Division is to identify correlations that we have not anticipated. In the case of cancer, for example, we compare cancer cells with normal cells or different subtypes of cancer. Usually, there are variables we would like to compare for analysis. However, there may not always be a correlation between the target variables, and there may be an unexpected correlation between other variables. The detection of such unexpected correlations requires a tremendous number of samples and analysis techniques that enable the processing of a large amount of data. We are currently accumulating such samples, and I would like to find new correlations and lead to the discovery of new truths in the future.
Between AI and humans, it does not mean that one is better than the other. Humans can find something subtle and assess it with their rules of thumb, whereas AI can analyze a large amount of data in an even-handed manner. A new science will surely be born if AI and humans work together with each other’s strengths. The same is true for other fields, and there must be something that can become visible for the first time by a collaboration between different fields. I think that it is the role of this Center to connect with various research institutes and make a network of various fields of research for such a future.
Tumor Pathology Yuzuru Ikehara
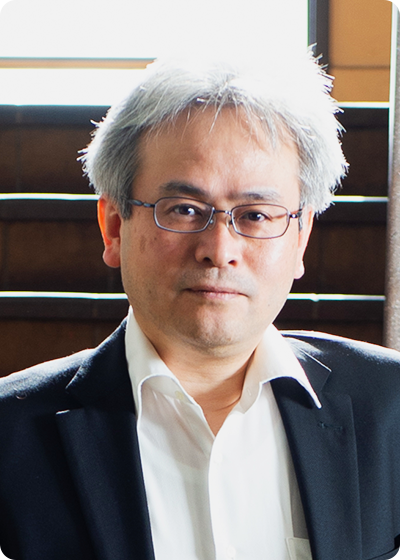
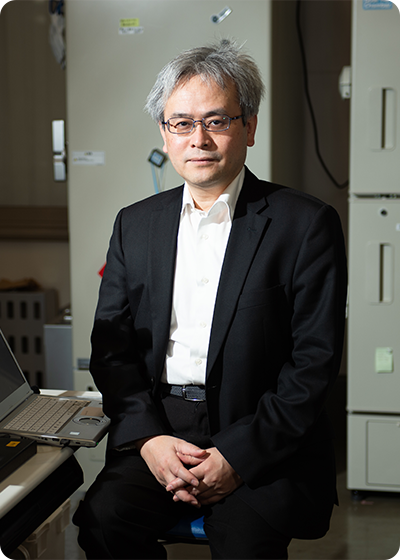
Yuzuru IkeharaProfessor, Department of Molecular and Tumor Pathology, Graduate School of Medicine, Chiba University
Senior Chief Scientist, Department of Life Science and Biotechnology, National Institute of Advanced Industrial Science and Technology
- Yuzuru IkeharaProfessor, Department of Molecular and Tumor Pathology, Graduate School of Medicine, Chiba University
Senior Chief Scientist, Department of Life Science and Biotechnology, National Institute of Advanced Industrial Science and Technology -
By the time a medical service becomes available for practical use, it has passed through a three-level hierarchical structure: the base-level hierarchy responsible for creating system design (pathology) and architectures (products); the middle-level hierarchy responsible for creating guidelines for using architectures and improving products to put them into practical use; and the top-level hierarchy where the established health services section plays a role as an actual working unit in managing the utilization of services and monitoring cost-effectiveness. Each level of hierarchy has different technical opportunities and different missions to be fulfilled, and therefore, there are different ways of using AI and different expectations and thoughts about AI itself. In this context, the Basic Research Division is in the middle-level hierarchy that is responsible for acting as a bridge to practical application for the base-level hierarchy. By utilizing AI, this Division works to “find an optimal solution that meets the needs of each phase,” that is, to optimize medical services.
One of the major roles of the Basic Research Division is to prepare a large amount of data for deep learning by AI. Using next-generation sequencers, we extract a large amount of data from samples brought in from each course, and provide the extracted data to the relevant course. For example, we sort cells, using a fluorescence-activated cell sorter (FACS machine), into more detailed types to see their expression and how the cells change over time after stimulation. Data obtained from samples may be provided as such to the relevant course, or we may support each course until the completion of analysis.
What the Basic Research Division expects from AI is to find correlations that we had not anticipated (overlooked). In the case of cancer, for example, cancer cells are diverse, and the supportive tissue called “tumor stroma” shows various forms even in a single patient due to its plasticity. For this reason, some information inevitably drops out of our consciousness during research where we roughly predict differences in genes and carbohydrate structures between cancer cells and precancerous cells in one patient, and verify and analyze the prediction. When we face a large amount of data, the effects of radiation and chemotherapy on the cells draw our attention, making the true correlation less noticeable, because normal cells grow more efficiently and are more conspicuous than cancer cells. We can expect AI to be able to reliably detect unexpected correlations even in these situations.
In addition, as the scope of research is expanded from individual patients to a population, the data will become even larger and the analysis more complex, making it difficult to find correlations. In fact, to reflect the patient’s clinical course, we try to obtain samples from a broad range of age groups, to ensure that the rates and numbers of smokers and drinkers are nearly equal between the target and control groups. However, this does not necessarily mean that we can successfully find a correlation.
To find an unexpected correlation, I presume that ten-thousands (≥10,000) of samples will be needed. Thus, we are now gradually accumulating such samples.
Since we make AI process this large amount of data, we believe that we will find new correlations in the future. To demonstrate this prediction, we have modified genes in genetically homogeneous mice to create a disease model of cancer, and are conducting the analysis. Even in the mouse model of carcinogenesis, the created cancer and tumor stroma show various histological pictures. By analyzing tumor tissue developed in the mouse model using AI, we believe that new discoveries will be generalized, leading to the creation of system design (pathology) and architectures (products). In this context, I hope that the links among new fields will become visible. I think that the role of this Center is to connect with various research institutions and make a network of various fields of research for such a future.
Medical AI Research Division
Artificial Intelligence (AI) Medicine Eiryo Kawakami
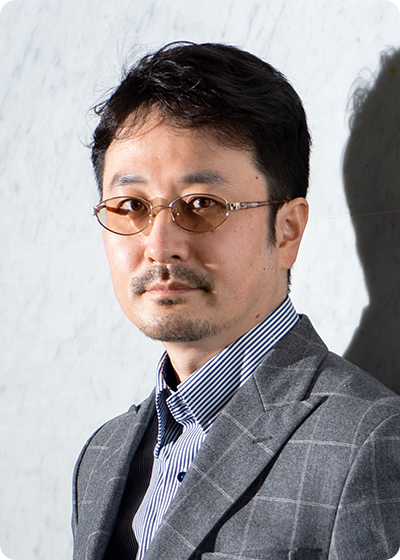
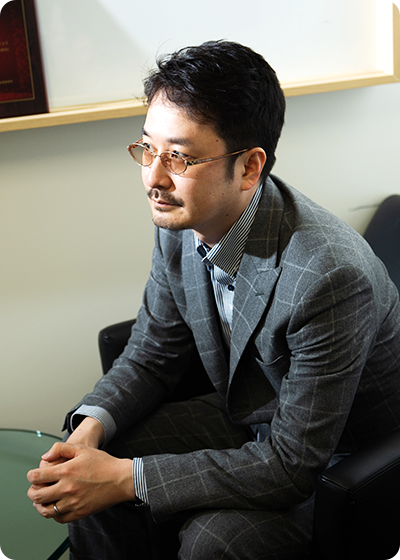
Eiryo KawakamiProfessor, Department of Artificial Intelligence Medicine, Graduate School of Medicine, Chiba University
Director of the Center for Artificial Intelligence Research in Therapeutics
RIKEN Medical Sciences Innovation Hub Program
Team Leader, Health Data Mathematical Reasoning Team
Unit Leader, Healthcare and Medical Data-Driven, AI-Based Predictive Reasoning Development Unit
- Eiryo KawakamiProfessor, Department of Artificial Intelligence Medicine, Graduate School of Medicine, Chiba University
Director of the Center for Artificial Intelligence Research in Therapeutics
RIKEN Medical Sciences Innovation Hub Program
Team Leader, Health Data Mathematical Reasoning Team
Unit Leader, Healthcare and Medical Data-Driven, AI-Based Predictive Reasoning Development Unit -
The Medical AI Research Division has two major themes. One theme is “change over time.” So far, we made a diagnosis and decided on a treatment approach at the time the patient visited our hospital. In other words, we looked at the patient only at that moment. We rarely used information on what clinical course the patient had taken. We are now studying changes of diseases over time, aiming to understand and predict how a disease develops and progresses, and preemptively prevent the disease before the occurrence of a major change.
The other is “redefinition of diagnosis.” Many multifactorial diseases, such as lifestyle-related diseases and cancer, cannot be clearly classified or diagnosed, and only an input of the existing disease classifications into AI does not lead to the improvement of diagnostic accuracy or treatment outcomes in many cases. By using a method called “unsupervised machine learning” in such cases, we are studying to find a clinically unnoticed disease classification in the data.
The other is “redefinition of diagnosis.” Many multifactorial diseases, such as lifestyle-related diseases and cancer, cannot be clearly classified or diagnosed, and only an input of the existing disease classifications into AI does not lead to the improvement of diagnostic accuracy or treatment outcomes in many cases. By using a method called “unsupervised machine learning” in such cases, we are studying to find a clinically unnoticed disease classification in the data.
Industry-Academia Collaboration Division
Emergency and Critical Care Medicine Takaaki Nakata
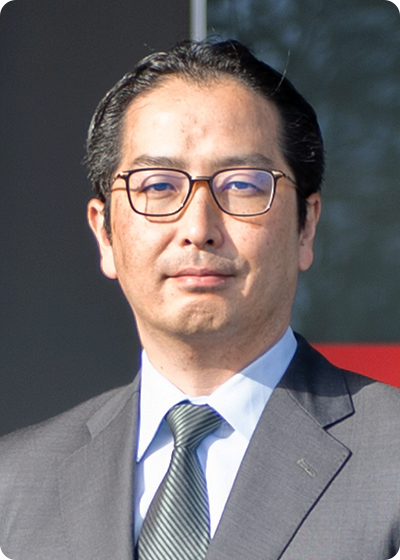
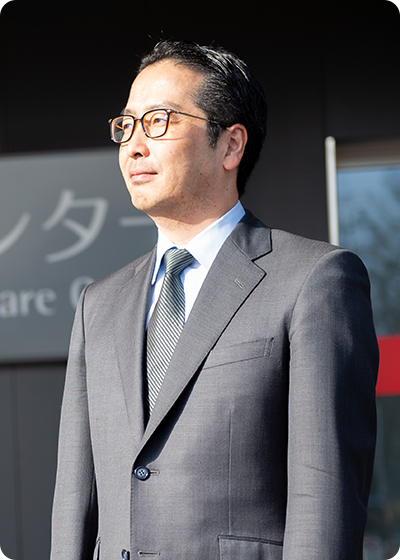
Takaaki NakataProfessor, Department of Emergency and Critical Care Medicine, Chiba University
Chief Executive Officer of Smart119, Inc.
- Takaaki NakataProfessor, Department of Emergency and Critical Care Medicine, Chiba University
Chief Executive Officer of Smart119, Inc. -
In May 2018, I established Smart119, Inc., Chiba University’s venture company. Our ICU has a database that has continued to store data every second, minute, and hour for the last 10 years. For example, we are inputting data from patients admitted to ICU into AI to determine the probability of surviving to hospital discharge, and analyze the algorithm that predicts when patients are weaned from ventilators. Our company has already provided multiple services, and our main service, “Smart119,” is a service for sharing patients’ information. Information entered using a tablet by a command center staff member and a paramedic can be seen on a personal computer (PC) or smartphone by healthcare providers at medical institutions, and this system has been introduced to Chiba City since July 2020.
In AI research, what is the most difficult is, in fact, to look for AI researchers, but our company has an advantage in that we have fortunately achieved it. We are continuing research, together with scientists who not only work in the AI field and but also are interested in healthcare. We hope that we will continue to provide services that enable companies and academia to collaborate well and contribute to the society. We also hope that young researchers interested in both AI and healthcare will be fostered here to make a brighter and better future for healthcare.
Educational System Development Division
Medical Education Shoichi Ito
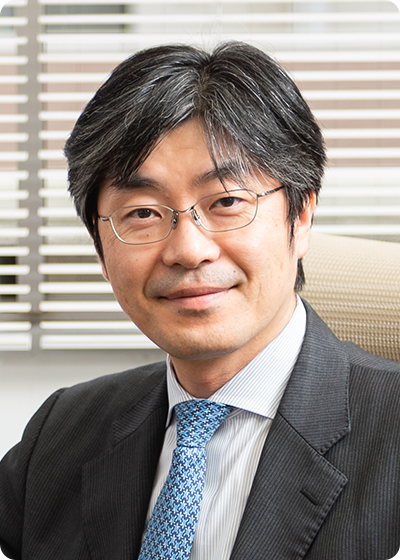
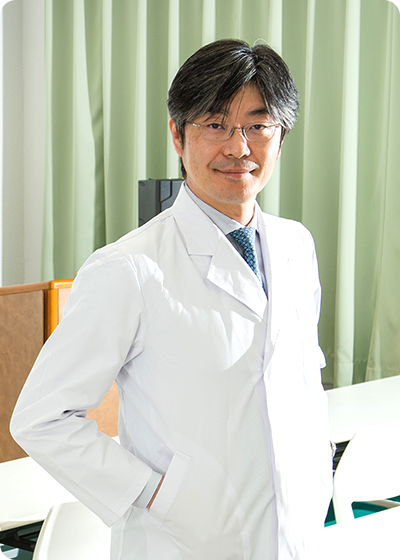
Shoichi ItoProfessor, Medical Education Office, Graduate School of Medicine, Chiba University
Head, Medical Education Office, Chiba University School of Medicine
Director, Health Professional Development Center, Chiba University Hospital
- Shoichi ItoProfessor, Medical Education Office, Graduate School of Medicine, Chiba University
Head, Medical Education Office, Chiba University School of Medicine
Director, Health Professional Development Center, Chiba University Hospital -
Currently, AI is becoming increasingly common in the healthcare setting. In the near future, it will probably be a thing taken for granted. We must foster “AI experts” if such a situation is realized, and it is the Educational System Development Division that is responsible for the fostering of AI experts. What is “AI human resources”? They are experts who have a good command of AI that continues to evolve at a tremendous speed, and who can always catch up with its evolution. In other words, we think that AI experts are human resources who can think flexibly from an interdisciplinary perspective while allowing all possibilities.
AI does not always propose a non-controversial hypothesis that may pop into the mind of humans. Therefore, I do not think that we can make the most of AI without a flexible, interdisciplinary attitude of accepting unexpected proposals without denying them. It is also important to have a feeling of working together with AI, instead of relying on AI, to solve healthcare challenges. Above all, what we need is the feeling of enjoying the challenges. Curiosity leads to motivation to learn and work, which will promote the development of both AI and healthcare.
To foster such AI human resources, we intend to create an educational environment that provides opportunities for learning but does not give too much detailed instructions. It is ideal for us to let students study freely after saying “there is an interesting issue” and providing information about the ground rules. In reality, it would be better for us to communicate the fundamentals in “AI Mathematical Sciences” in the universal (general) education course of Chiba University and provide interested students with opportunities to study under the direction of a professor. I hope that we will be able to foster as many AI experts as possible who can identify what AI is good and poor at, and work together with AI to solve healthcare challenges.
ACCESS &
CONTACT
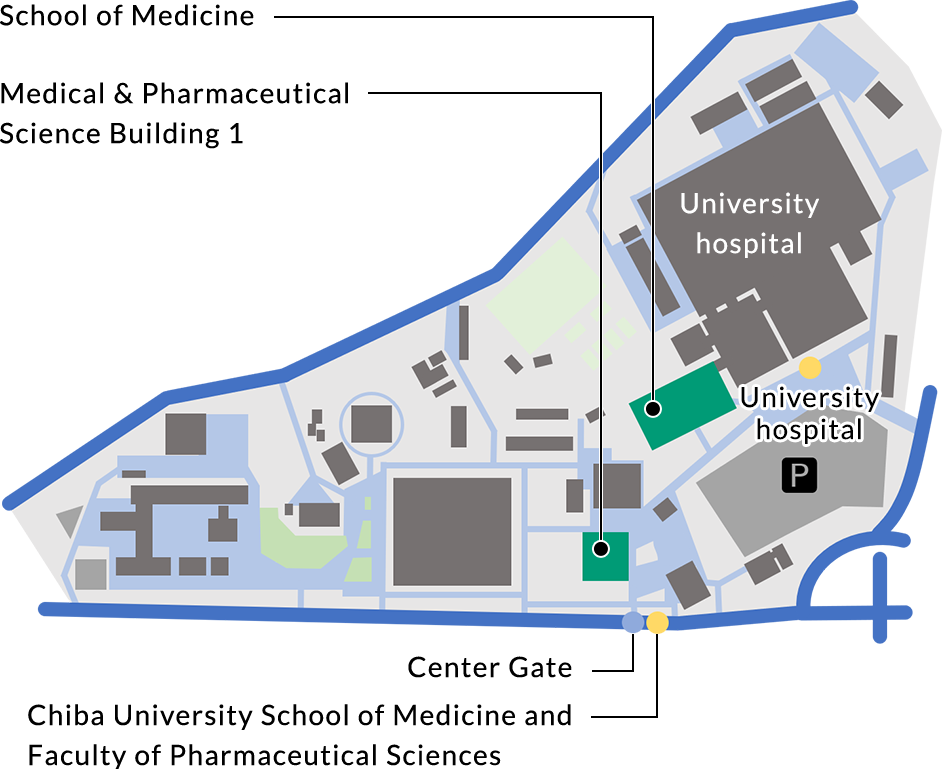
ACCESS
- From JR Chiba St.
- Service route: Chiba University Hospital/Minami Yahagi from Bus stop No.7 at east exit.
Operator: Keisei Bus.
Duration: approx. 15 min.
Get off at “Chiba University School of Medicine and Faculty of Pharmaceutical Sciences”.
- From JR Soga St.
- Service route: University Hospital from the Bus stop No.2 at east exit.
Operator: Kominato Bus/ Chiba-chuo Bus
Duration: approx. 13 min.
Get off at “University Hospital”.
CONTACT
5F, School of Medicine, 1-8-1
Inohana, Chuo-ku, Chiba-shi,
Chiba
260-8670, JAPAN
Department of Artificial Intelligence Medicine
E-mail: keiko-y@chiba-u.jp